Climate Change Analysis Based on Satellite Multispectral Image Processing in Feature Selection Using Reinforcement Learning
DOI:
https://doi.org/10.17762/ijcnis.v14i2.5520Keywords:
Reinforcement learning, Satellite images, Climate change, Deep learning, Parameter selectionAbstract
Currently private and government agencies use remote sensing images (RSI) for various applications from military applications to agriculture growth. The images can be multispectral, panchromatic, ultra-spectral, or hyperspectral of terra bytes. RSI classification is considered one important application for remote sensing. Climate change detection especially affects numerous aspects of day-to-day lives, for instance, forestry management, weather forecasting, transportation, agriculture, road condition monitoring, and the detection of the natural atmosphere. Conversely, certain research works had a focus on classification of actual weather phenomenon images, generally depending on visual observations from humans. The conventional artificial visual difference between weather phenomena will take more time and error-prone. This paper develops a new reinforcement learning based climate change analysis on satellite multispectral image processing (RLCCA-SMSIP) technique. In order to properly determine climate change, the RLCCA-SMSIP technique employs residual network (ResNet-101) model for feature extraction. Next, deep reinforcement learning (DRL) approach is utilized for climate classification. Finally, parameter selection of the RLCCA-SMSIP technique involves sine cosine algorithm (SCA) for DRL model. For assuring the enhanced outcomes of the presented RLCCA-SMSIP model, comprehensive comparison results are assessed. The obtained values denote the supremacy of the RLCCA-SMSIP model on climate classification.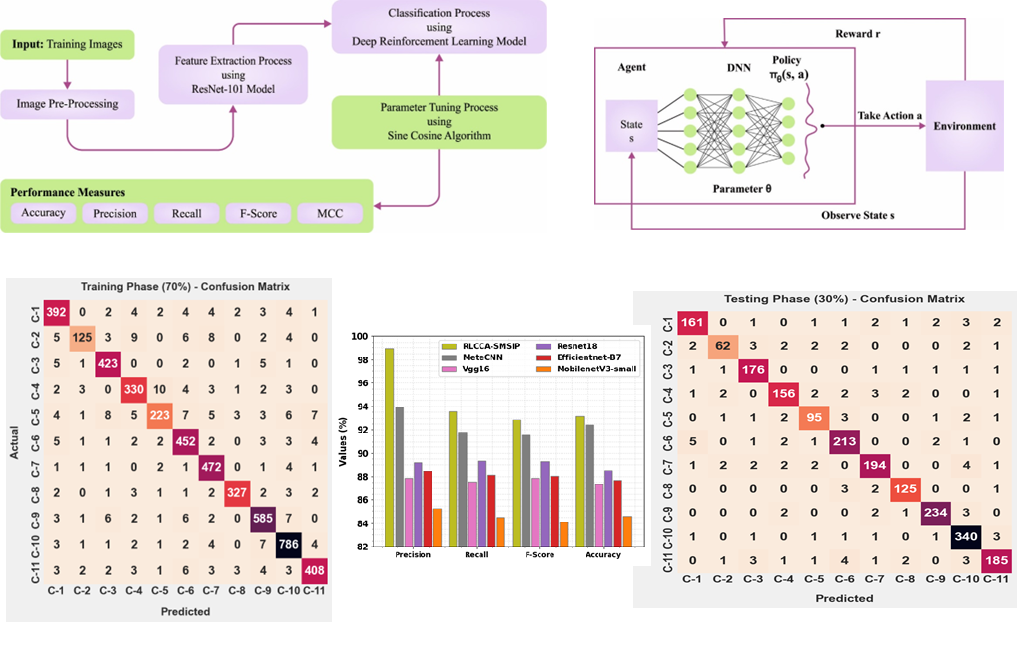
Downloads
Published
How to Cite
Issue
Section
License
Copyright (c) 2022 Author

This work is licensed under a Creative Commons Attribution-NonCommercial-ShareAlike 4.0 International License.