Restrictive Voting Technique for Faces Spoofing Attack
DOI:
https://doi.org/10.17762/ijcnis.v15i1.5738Keywords:
Machine-learning, Face Anti-spoofing, SVM, LBP, Voting Techniques, Majority VotingAbstract
Face anti-spoofing has become widely used due to the increasing use of biometric authentication systems that rely on facial recognition. It is a critical issue in biometric authentication systems that aim to prevent unauthorized access. In this paper, we propose a modified version of majority voting that ensembles the votes of six classifiers for multiple video chunks to improve the accuracy of face anti-spoofing. Our approach involves sampling sub-videos of 2 seconds each with a one-second overlap and classifying each sub-video using multiple classifiers. We then ensemble the classifications for each sub-video across all classifiers to decide the complete video classification. We focus on the False Acceptance Rate (FAR) metric to highlight the importance of preventing unauthorized access. We evaluated our method using the Replay Attack dataset and achieved a zero FAR. We also reported the Half Total Error Rate (HTER) and Equal Error Rate (EER) and gained a better result than most state-of-the-art methods. Our experimental results show that our proposed method significantly reduces the FAR, which is crucial for real-world face anti-spoofing applications.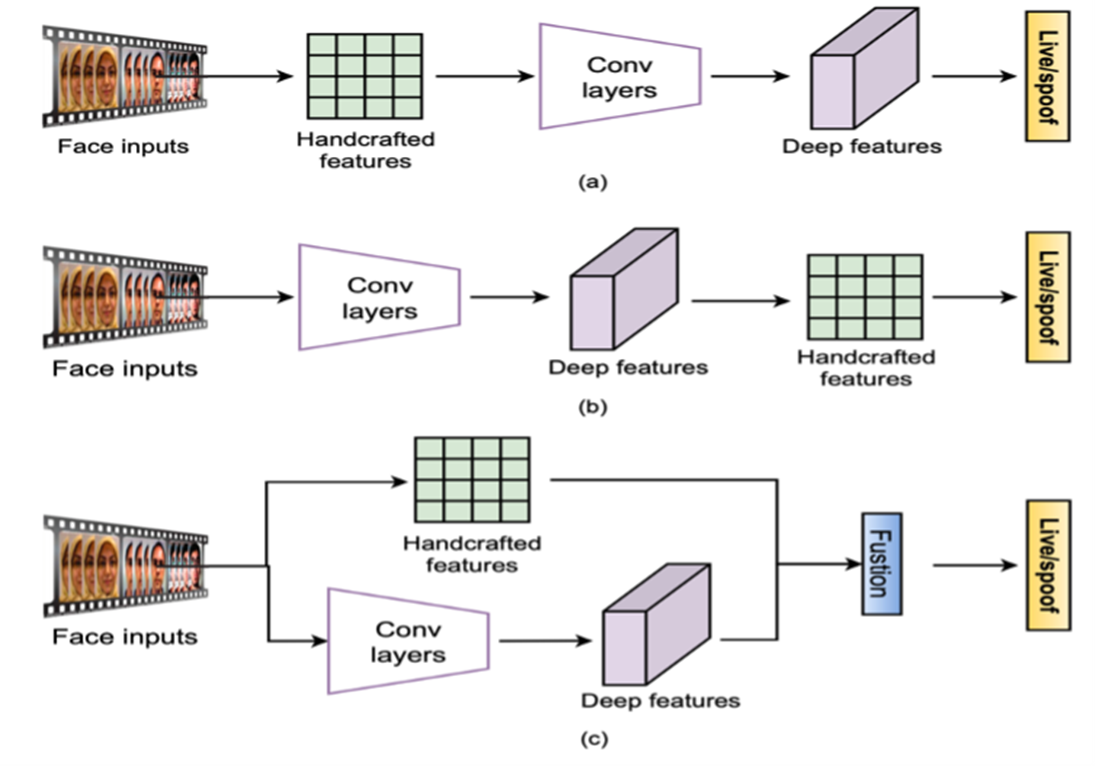
Downloads
Published
How to Cite
Issue
Section
License
Copyright (c) 2023 International Journal of Communication Networks and Information Security (IJCNIS)

This work is licensed under a Creative Commons Attribution-NonCommercial-ShareAlike 4.0 International License.