Hybrid Intrusion Detection Model for Enhancing the Security and Reducing the Computational Cost
DOI:
https://doi.org/10.17762/ijcnis.v15i2.6188Keywords:
Cybersecurity, Intrusion Detection System, Decision Tree, Linear Regression, Feature Reduction, AdaBoost Technique, Computational CostAbstract
Artificial Intelligence (AI) is becoming essential technology in Cybersecurity. It represents a revolution in detecting and analyzing intrusions based on predictive models and classification methods. Various recent studies discussed the applications of artificial intelligence in Intrusion Detection Systems to improve the accuracy of the classifiers in detecting cyber-attacks but ignored the computational cost of running the algorithm which is considered a crucial factor of the model evaluation. The aim of this paper is to solve this security issue by using dimensionality reduction techniques and machine learning algorithms. To raise their effectiveness and thus enhance network security, a hybrid classifier with high accuracy and low computational cost is proposed. It combines Decision Tree (DT) and Linear Regression (LR) techniques with AdaBoost technique to build a powerful model for detecting cyber-attacks. The hybrid model included 5 stages, (i) selecting and analyzing the dataset, (ii) pre-processing it, (iii) reducing the dimensions using the Principal Component Analysis (PCA), (iv) classifying stage and (v) evaluating the model using the dataset UNSW-NB15. The model has been compared with several state-of-the-art algorithms. The results have shown that the proposed hybrid model achieved a high accuracy (99%) and the runtime was significantly reduced by half using PCA principle.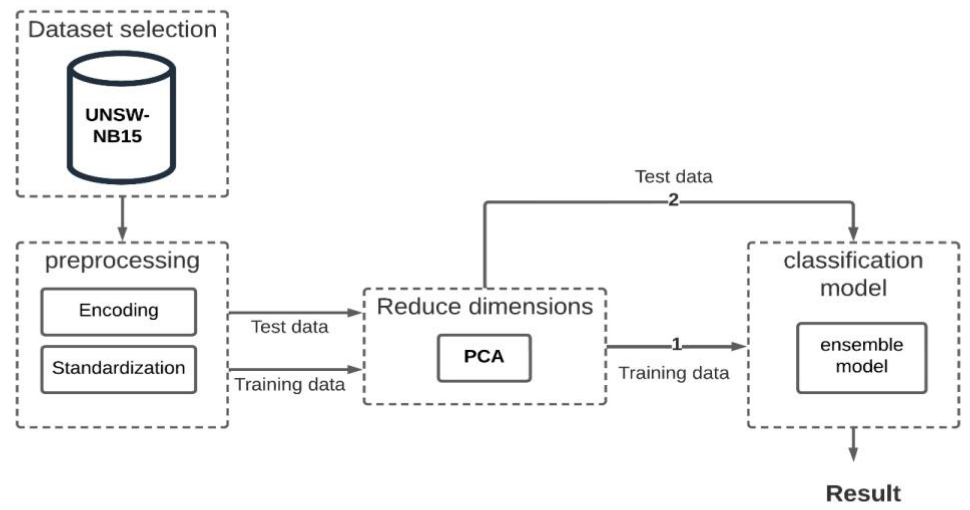
Downloads
Published
How to Cite
Issue
Section
License
Copyright (c) 2023 International Journal of Communication Networks and Information Security (IJCNIS)

This work is licensed under a Creative Commons Attribution-NonCommercial-ShareAlike 4.0 International License.