Design of an Integrated Model for Security Establishment in Iot-Enabled Software Defined Networks
DOI:
https://doi.org/10.17762/ijcnis.v16i1.6466Keywords:
Cyber Security, Prediction, Attack, Optimization, Machine LearningAbstract
Robust network designs are provided by software-defined networks (SDNs) for Internet of Things (IoT) applications, both present and future. At the same time, because of their programmability and global network perspective, SDNs are a desirable target for cyber threats. Among its primary drawbacks is the susceptibility of standard SDN architectures to Distributed Denial of Service (DDoS) flooding attacks. DDoS flooding assaults often result in a complete failure or service outage by rendering SDN controllers useless with respect to their underlying infrastructure. This study looks at popular machine learning (ML) methods for classifying and detecting DDoS flooding attacks on SDNs. Restricted Boltzmann Machine with Restricted Whales’ Optimizer (RBM-RWO) is the classifier integrated optimizer and other machine learning techniques examined. In this case study, experimental data (jitter, throughput, and reaction time measurements) from a realistic SDN architecture appropriate for typical midsized enterprise-wide networks are used to construct classification models that effectively detect and describe DDoS flooding assaults. Attackers using DDoS floods used low orbit ion cannons (LOIC), user datagram protocol (UDP), transmission control protocol (TCP), and hypertext transfer protocol (HTTP). Despite the high effectiveness of all the ML techniques examined in identifying and categorizing DDoS flooding assaults, When it came to training time is 17.5 ms, prediction speed is 7e-3 observations/s, prediction accuracy of 98%, and overall performance, RBM-RWO performed the best.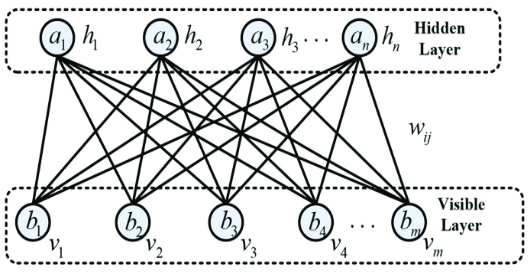
Downloads
Published
How to Cite
Issue
Section
License
Copyright (c) 2024 International Journal of Communication Networks and Information Security (IJCNIS)

This work is licensed under a Creative Commons Attribution-NonCommercial-ShareAlike 4.0 International License.